Interview with Goran Georgievski, modeler in the MOMENT project
19 March 2025, by Christina Steffens
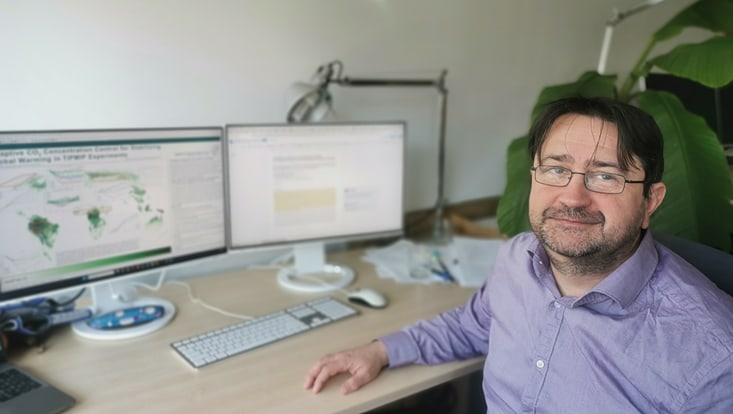
Photo: UHH/C.Steffens
Dr. Goran Georgievski is a researcher at the Max Planck Institute for Meteorology within the “Climate-Biosphere Interactions” working group lead by Prof. Dr. Victor Brovkin. Trained as a physical engineer specializing in meteorology and physical oceanography, Georgievski has acquired significant expertise in data analysis, high-performance computing, and numerical modeling over his scientific career at various German research institutions. His proficiency lies in integrating in-situ observations and remote sensing data with advanced climate models. Currently engaged in the MOMENT project, we aim to delve into his research and gain interesting insights during this interview.
Please click on the topic below you are interested in to activate the corresponding part of the interview.
MOMENT work
Hello Goran! What is your research question in the MOMENT project?
Hi Christina, and thank you for this opportunity to share some insight and provide more information on my research. The major objective in the modelling subproject of MOMENT is to reduce uncertainty in regional pan-Arctic methane (CH₄) emissions for the historical period and to estimate the potential range of CH₄ emissions in a warming world as permafrost thaws. Our key research questions focus on understanding how environmental conditions—such as soil temperature, water availability, and oxygen stress—affect microbial activity that regulates the decomposition of carbon from thawed soil, and how these factors will influence the amount of carbon dioxide (CO₂) and CH₄ emitted into the atmosphere.
What parameters are you currently working on to better map the methane balance in the permafrost regions?
In our model, the parameters controlling CH₄ production and the ratio between produced CO₂ and CH₄ under anaerobic conditions are based on highly uncertain empirical or semi-empirical relationships. The Q10 factor is identified as a key parameter for calibration to improve CH₄ emission maps. In collaboration with our project partners from Jena (Max Planck Institute for Biogeochemistry), we are using inverse atmospheric modelling technique to evaluate the impact of different Q10 values on CH₄ wetland fluxes.
How does it work?
The method is as follows: we provide wetland CH₄ emissions data from the JSBACH model, as input (prior flux) for atmospheric inverse modelling i.e. CarboScope model used by our partners in Jena. Their model also incorporates other sources of CH₄, which are fed into an atmospheric CH₄ transport model. The inverse model optimizes the total CH₄ fluxes by adjusting them to best match atmospheric observations. By comparing the necessary adjustments for each Q10-based wetland flux, we determine which Q10 value results in the smallest flux adjustment, indicating the best agreement with the observed data. A major challenge is the scarcity of observation towers in the Arctic region and limited temporal coverage.
What does the Q10 factor tell us?
The Q10 factor generally quantifies the impact of a 10°C increase in temperature on the speed of a chemical reaction. In our case, related to wetland CH₄ production, it controls how a temperature change of 10°C modifies ratio of carbon decomposed by heterotrophic respiration into CO₂ and CH₄.
The Q10 factor is strongly variable among ecosystems also within one climate region. How do you deal with it?
Indeed, the Q10 factor is traditionally viewed as constant for most biological reactions, typically ranging from 2 to 3. However, recent studies suggest that a constant Q10 coefficient may not accurately capture the temperature sensitivity of organic matter decomposition. Consequently, as mentioned earlier, we employ inverse modelling techniques to refine and narrow the range of plausible Q10 values.
Work with JSBACH
Earlier you mentioned the model JSBACH, which you use? Can you explain in simple terms what this model does?
Yes, I am using JSBACH, the land component of the Max Planck Institute for Meteorology Earth System Model (MPI-ESM). The atmospheric component of MPI-ESM solves equations to determine atmospheric circulation patterns, but also environmental conditions at the atmosphere-land interface, such as surface temperature, precipitation, albedo, surface roughness, and snow cover. These are all relevant for Earth’s energy balance, carbon and hydrologic cycle. Alternatively, these surface conditions can be prescribed from observed climatic parameters for more realistic estimates. In both cases, JSBACH solves the equations governing heat and moisture propagation into soil layers. Additionally, it features a vegetation module that sequesters CO₂ from the atmosphere, along with modules for soil carbon decomposition (i.e. soil heterotrophic respiration).
That sounds like a quite complex model. Soil carbon decomposition strongly depends on several soil physical and biochemical properties, as well as soil climate. How is that taken into account?
It may sound complex indeed, and it certainly is in the nature. However, have in mind that we are talking about a model, which serves as a simplified representation of the complex process actually occurring in nature. The rate of carbon decomposition in JSBACH is influenced by temperature, water availability, and litter size. It increases exponentially with rising temperatures and decreases asymptotically to zero as temperatures drop below the melting point. Water availability affects decomposition rates, increasing exponentially from zero in dry conditions to one in water-saturated conditions. Litter size also plays a role, with decomposition rates declining asymptotically from one to zero as litter size increases.
And how about soil parameters such as soil acidity? Is land-use also considered?
JSBACH differentiates five distinct soil carbon pools- acid hydrolysable, water soluble, ethanol soluble, neither hydrolysable nor soluble, and humus- based on their solubility and chemical reactivity. These pools are replenished by decomposing vegetation. Apart from the physical conditions already described, the decay of soil organic carbon in each pool is also regulated by an empirically determined baseline decomposition rate constant, which depends on both solubility and chemical reactivity. Changes in land cover due to land use are also considered, by prescribing maps of vegetation change that affect biophysical properties (e.g., albedo, surface roughness, transpiration potential), as well as associated relocations of carbon and nutrients between the different pools and to the atmosphere.
Thank you! Back to the Arctic. What else needs to be considered?
In the permafrost regions of high northern latitudes, wetlands are the main natural source of CH₄. To identify inundated (wetland) areas, a rainfall–runoff model based on the compound topographic index (CTI) is used. These areas are characterized by anaerobic (low oxygen) conditions that enhance microbial CH4 production. CH₄ production is influenced by the Q10 coefficient, which governs temperature sensitivity and regulates the proportions of CO₂ and CH₄ during carbon decomposition.
About TIPMIP and tipping elements
You've been to a few congresses and workshops in the last year, one of them was the TIPMIP General Assembly. What did you present there?
Yes, I participated in the Tipping Point Model Intercomparison Project (TIPMIP) General Assembly in Baltimore. There, I presented a comparison between adaptive CO2 concentration-driven MPI-ESM simulations and adaptive CO2 emission-driven MPI-ESM simulations. Both simulation sets were designed to stabilize global warming at levels of 2°C, 3°C, and 4°C above pre-industrial global mean temperatures, with a focus on tipping elements in the climate system.
What are tipping elements in the climate system?
Tipping elements are characterized by nonlinear dynamics in a system where small perturbations or gradual changes in forcing (e.g., redistribution of carbon in the Earth System) trigger disproportionately large, often abrupt, and potentially irreversible shifts in the system’s state. Examples of tipping elements can include melting of polar ice caps, changes in ocean circulation patterns, release of permafrost carbon or the dieback of forests. Once these systems are tipped, they can cause cascading effects throughout the climate system.
Is permafrost thaw also a tipping element?
Whether permafrost thaw represents a tipping element remains a subject of debate, likely depending heavily on the specific definition of a tipping element. However, one of the factors on which depends if permafrost exhibits tipping or not depends on how much carbon will be released into the active layer and how much of it will be decomposed as CO2 and how much as CH4.
Thank you for this small excursion. Let`s come back to the content of your presentation at the TIPMIP General Assembly.
Sure. The primary focus of the research that I presented was to assess the potential impact of permafrost carbon within a warming climate and how this might affect the remaining carbon budget necessary to maintain temperature below established warming limits, which also contributes to MOMENT project objectives. In our simulations by the year 2300, the average rate of permafrost carbon release is projected to be between 0.3 and 0.7 PgC/yr under global warming scenarios of 2°C and 3°C, respectively. This represents 3-7% of the current anthropogenic fossil fuel emissions of 10 PgC/yr. While this may seem small in the context of present-day fossil fuel emission, it is significant in the context of achieving net-zero emissions.
What was your main take-home message from the meeting?
The main takeaway from the meeting was that model responses tend to be linear and struggle to accurately represent the nonlinear processes that characterize tipping elements.
How trustworthy are then the model outcomes of future scenarios that probably cause tipping of an element, such as a 4°C warming scenario?
Earth System Models have substantial constraints in representing natural feedbacks, for example, those linked with greenhouse gas emissions resulting from permafrost thaw, or impacts on continental shelf circulation caused by freshwater runoff from ice sheet melting. Related to permafrost thaw, the ratio of CO2 to CH4 emissions into the atmosphere remains largely unknown, as does the corresponding climate feedback. Current models lack resolution and processes representation to capture spatial heterogeneity in thaw dynamics (e.g., abrupt thermokarst formation versus gradual thaw) or microbial pathways (e.g., methanogenesis under varying environmental conditions). These uncertainties undermine our ability to quantify the permafrost feedback’s true magnitude or nonlinearity, risking underestimation of its role in climate tipping dynamics. On the other hand, your question regarding the reliability of models, is rather philosophical one. Models are thought experiments on computers, representing theoretical simplification of our understanding of reality. They are as good as our understanding of reality, provided that the technical implementation of this understanding in the computer code is free from bugs.
Interesting. So it means that much more field research is needed to improve our understanding of reality.
Back to MOMENT work - final questions
We learned from the field researchers in the MOMENT project that the methane fluxes on Greenland's Disko Island vary significantly on a small spatial scale and exhibit strong seasonality. However, the resolution in the Earth system models is much greater. How do you deal with small-scale variations in the models?
Bridging the gap between local-scale processes and estimating their regional and global impacts is a major challenge and an active area of research and model development. MPI-ESM and JSBACH are global models and operate at coarse resolutions of about 200 km. However, for specialized studies, we can run JSBACH at smaller scales or even at the site level, though obtaining reliable climatic forcing and initial conditions becomes more difficult in these cases. To capture fine-scale processes not resolved by coarse models, we often rely on subgrid parameterizations based on statistical methods. For example, the Q10 parameter mentioned earlier is assigned a single, constant global value in our simulations, typically derived from some form of regression analysis. Recent studies, however, shows that Q10 largely varies depending on microbial community composition, temperature, and other local environmental factors. Consequently, adjusting Q10 to account for its spatial and potentially seasonal dependencies could substantially improve our wetland CH₄ flux estimates. Therefore, the answer to your question is that we do not resolve microbial communities explicitly in our model, but with the bulk value of Q10 for the whole grid we approximately estimate effect of microbial activity.
One last question: What do you particularly like about the MOMENT project?
I like the collaboration within our subproject group and the project meetings. They are highly productive and provide an excellent opportunity to learn and gain insights, especially from colleagues doing in situ observations. It would be wonderful if at least one of these meetings could be organized on Disko Island, allowing us modelers the opportunity to visit the observation sites.